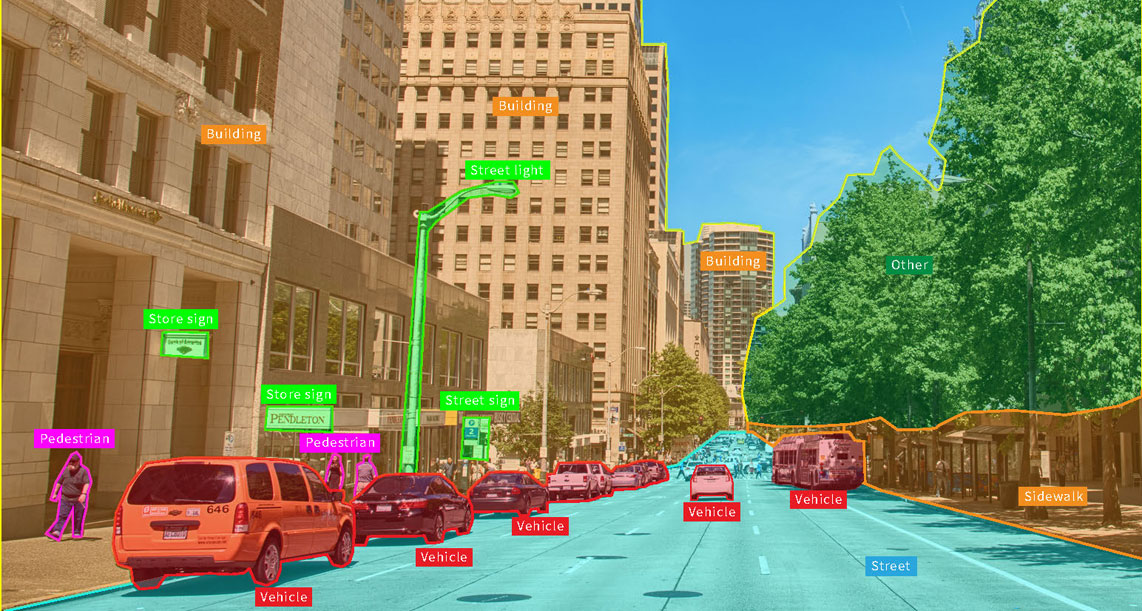
Chances are you’ve interacted with object recognition technology before, without even realizing it.
From alerting about a supply chain irregularity to identifying potential threats in public spaces, object recognition has become increasingly present in our day to day. This technology helps us analyze, understand and react to the things around us. However, object recognition is especially critical in security, where discerning an inoffensive object from a harmful one can be the difference between safety and disaster.
This article explores how object recognition is reshaping our understanding and approach to security in today’s world. Plus, it discusses how advancements in artificial intelligence (AI) are driving the field forward.
Table of contents
- The basics of Object Recognition: What is it and how does it work?.
- Types of Object Recognition.
- Object Recognition vs. Object Detection.
- 3 Benefits of Object Recognition in Security Systems.
- How AI and Machine Learning have transformed object recognition.
- What are the challenges of Object Recognition?.
- Future Trends and Expected Evolution of Object Recognition.
The basics of Object Recognition: What is it and how does it work?
Object recognition is a technology that allows machines to understand, recognize, and detect the contents of an image or video.
Thanks to advancements in AI, this tool has seen rapid development, finding its way into various sectors, such as security or retail as well as in airports, self-driving cars, and even social media.
The basics of how object recognition functions are:
- Images or videos are captured, often in real-time, and fed into systems trained with Machine Learning or Deep Learning algorithms.
- These systems then process the visuals, basing their interpretations on several factors depending on what they are trying to detect, why, and with how much accuracy or specificity. for example, the size of the objects, the shape, the color, the movement, their distance in relation to other objects, etc.
Furthermore, object recognition allows computers to detect, label, and organize specific objects within the images and footage it is implemented to.
An everyday use for this would be photograph apps such as Google Photos, which is capable of detecting faces but can also discern between dogs, cats, buildings, menus, documents, books, and plenty of other objects.
Furthermore, Google Photos labels these photographs and creates categories for these objects or for particular people. However, this technology has also been implemented in airports or public spaces to help detect objects like weapons (firearms or knives) or suspicious packages. Other examples of their use are the face unlock feature of several phones or computers and the object recognition of self-driving cars, which allows them to detect other cars, pedestrians, animals, or other information relevant to the proper functioning of the car.
In the following sections, we will explore how object recognition works and how it can be useful in our society.
3 COMMON APPLICATIONS OF OBJECT RECOGNITION
Types of Object Recognition
Object recognition technology, while often discussed as a singular entity, encompasses a series of computational tasks. Each task plays a distinct role in interpreting an image or video frame. We will briefly explain the most important of these: image classification, object localization, and image segmentation.
IMAGE CLASSIFICATION
The simplest task (in theory, although not in practice) in the object recognition process is image classification, which allows the algorithm to assign a class label to an image. In other words, it is the first filter that labels an image as, for example, a cat image, a portrait of a person, or a picture of a car.
In this part of the process, it is not clear yet where the object in the image is exactly, but the object is there, and thus, the image is labeled and placed in the category of images that show that object. In some cases, the algorithm can also be trained to detect if an object is not there.
OBJECT LOCALIZATION
Elevating the process further, object localization not only identifies the primary object but also delineates its position in the image with a bounding box. This box provides the position, height, and width of the object within the image and its relation to other objects.
OBJECT DETECTION
Object Detection requires both Object Localization and Image Classification since it creates bounding boxes for each object in the picture and labels them with a class. This allows for multiclass classification of a single image, which could be useful when searching images of each object or when trying to find images that contain two or more specific objects together.
However, it must be said that in object detection, the bounding boxes are always rectangular, meaning that the shape of the objects is still unknown to the computer, making it unable to measure, for example, the area of an object or its perimeter.
IMAGE SEGMENTATION
This task consists of the marking of the recognized objects by highlighting the pixels that make it. This creates a mask over the object which can help detect the shape of the object and its actual dimensions in relation to the rest of the image.
This is a more advanced task than object detection and it requires more specialized and complex algorithms, but it is pivotal for some fields (for example, for medical image processing, in which the shape and dimensions of some elements are essential for an accurate diagnostic).
Object recognition vs. image recognition
It might be relevant to talk about another concept if only to avoid confusion: image recognition. It sounds similar to ‘Object recognition’ which is the technology we have been discussing so far, but there are some relevant distinctions between these two concepts.
Image Recognition
This process involves identifying and categorizing an entire image based on its predominant content. For instance, an image featuring an airport departure lounge would simply be categorized under 'airport lounge'. It provides a broader, holistic view of an image.
Object Recognition
Contrastingly, object recognition offers a granular analysis. Utilizing the same airport image, this technology would individually identify and categorize every discernible object, such as passengers, luggage, and possibly even specific brands of items.
This does not mean that one model is better than the other, but rather that each of these has specific uses and is better implemented in certain situations. Image recognition, for example, is amply used by social media such as Facebook, Google, and Pinterest, in which images are simply labeled and classified. However, Object recognition is useful in matters of security such as vehicle and people detection, tracking objects, and surveillance in general.
3 Benefits of Object Recognition in Security Systems
We have suggested before that Object recognition has been implemented in Security Systems to enhance the functionality of the system and ensure the safety of people or valuable objects.
In this section, we will discuss three ways in which these systems can benefit from the use of object recognition both in the public and private sectors:
Real-time detection and rapid responses
The use of live-feed cameras has become one of the necessities for houses, stores, banks, airports, malls, and even streets and highways. When enhanced with object recognition, the footage collected can be analyzed in real-time by the algorithm and flags can be sent to the system whenever a weapon or suspicious object is detected.
The help of computer vision in security allows for rapid responses by security guards, police officers, or other people in charge, depending on the situation, and helps reduce safety risks.
Reduction of false positives
The effectiveness of security walls monitored exclusively by humans is affected by several factors, such as attention span, exhaustion, and ability to multitask, among others. Furthermore, these factors can result in false positives regarding the detection of suspicious activity, weapons, abandoned packages, or other safety hazards in public and private places.
A well-trained AI, enhanced with computer vision and object recognition, can work together with security guards monitoring the footage to reduce possible false positives, which ultimately saves valuable resources for the companies. This is done by effectively tagging and signaling the objects in an image by using a bounding box that can even follow a movable object. The AI will only send an alarm if a dangerous object has been accurately recognized, which the human will be able to corroborate immediately.
Integration with other systems and technologies
Systems enhanced with Object recognition technology do not stand alone when ensuring the safety of people or valuable objects. A more complex and functional system can be created by integrating object recognition with other systems and technologies, such as movable cameras, alarms, electronic gates, smart lights, or telephone lines. This connection can be done via the internet, which ultimately helps to keep all the information updated and at hand.
For example, if a trespasser is detected in a certain area, the movable cameras can follow the suspect using object recognition, while at the same time, an alarm can be triggered, the gates shut, and the police department contacted automatically. These complex surveillance systems use cutting-edge technology to prevent crimes or at least catch the criminals as fast as possible. Systems like these have been implemented both in the private sector and in the public sphere, where police departments use them to keep the citizens safe and to keep criminals off the streets.
3 BENEFITS OF AN OBJECT RECOGNITION IN SECURITY SYSTEMS
How AI and Machine Learning have transformed object recognition
Artificial Intelligence and Machine Learning, especially deep learning techniques, have been pivotal in the meteoric rise and transformation of object recognition. Prior to these advancements, traditional image processing techniques struggled with scalability and adaptability.
These are some of the ways in which AI and ML have impacted object recognition technologies.
- Deep Learning Models: Neural networks, specifically Convolutional Neural Networks (CNNs), have shown unparalleled efficacy in image-related tasks. By simulating a human's ability to "learn" from data, these networks automatically extract features from images that are most pertinent for object recognition.
- Large Datasets: AI and ML models thrive on vast amounts of data. Platforms like ImageNet, with millions of annotated images, have allowed models to understand the intricacies of diverse objects under varying conditions.
- Transfer Learning: AI models trained on one task can now be adapted for another related task with minimal changes. This means models trained on general object recognition can be fine-tuned for specific tasks, like identifying medical images or detecting specific objects in security footage.
If you want to learn more about how Artificial Intelligence works, read our complete guide here.
What are the challenges of Object Recognition?
As object recognition technology continues to mature and integrate into diverse sectors, it's of crucial importance to understand the difficulties that developers and regular users might face.
These challenges will share the current landscape but also inform future advancements. Here are some of the most important challenges of object recognition:
- Privacy Issues and Regulations: As object recognition becomes pervasive, concerns about privacy intrusion have grown. Regulations like the General Data Protection Regulation (GDPR) in the European Union are setting strict rules about data collection and processing.
- Current Technical Limitations: Object recognition can struggle in certain situations due to poor lighting, overlapping objects, or similar-looking objects. These limitations can lead to misidentifications or missed detections. It’s important that users and buyers alike are aware of the limitations of the products they acquire and utilize.
- Importance of Regular Updates and Continuous Training: Like any AI system, object recognition models can become outdated. New objects emerge, and existing objects might change in appearance. Constant training and updating of these models are essential to ensure accuracy and relevance. However, it also implies a constant investment of time and effort.
As we navigate these issues, it becomes equally crucial to cast an eye to the horizon, anticipating the next wave of developments in the realm of object recognition.
Future Trends and Expected Evolution of Object Recognition
As it has been laid out in this article, the future of object recognition looks very promising. The most recent trends hint at what the future holds and how technology might seamlessly weave into our daily lives.
Here are some of the ways object recognition is shaping our future:
- Integration with Augmented Reality and Virtual Reality: As AR and VR technologies become mainstream, object recognition will play a pivotal role in merging digital and physical realities.
- Self-learning Systems: Future object recognition systems might not need vast labeled datasets. Instead, they could learn in an unsupervised or semi-supervised manner, making the training process more autonomous and efficient.
- Domain-specific Solutions: As the technology matures, we will see more specialized solutions catering to niche areas, whether it's recognizing rare species in deep-sea exploration or aiding archaeological discoveries.
The horizon for object recognition is vast, and as technology advances, its influence on our lives is set to grow even more profound.
vehicleDRX, our video analytics product with AI propelling the productivity of command centers, leverages object recognition technology to its maximum to monitor suspicious behavior, while also finding and tracking any vehicle of interest. Learn more about it here.